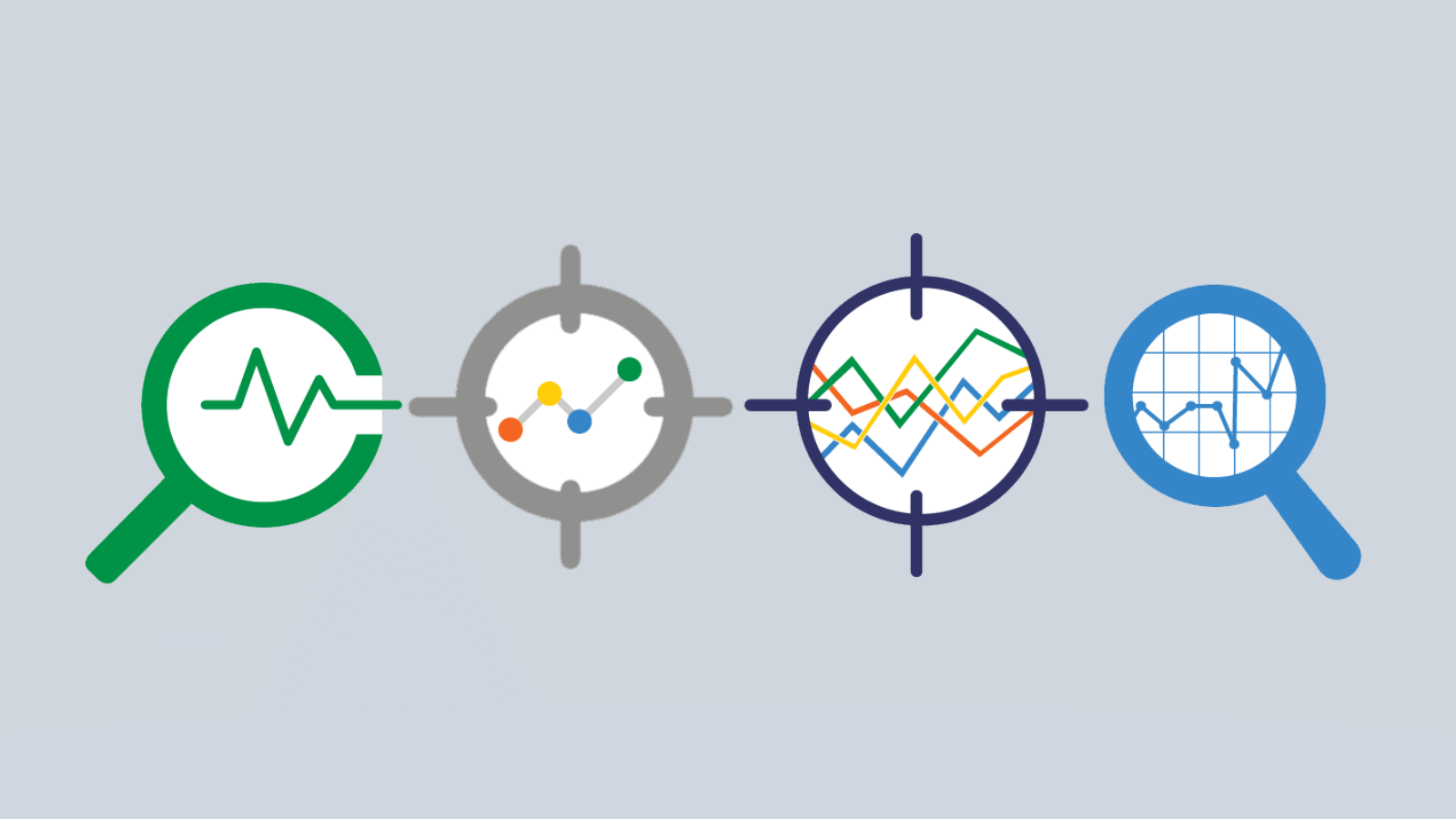
CFOs, Treasurers and their teams are increasingly looking at more intelligent and efficient ways to manage business on a day-to-day basis. One of the areas that is constantly in focus for improvement is cash flow forecasting which, though it is a business-critical activity, has historically been time consuming and inaccurate.
Because of the enormous potential for improvement in cash forecasting accuracy and efficiency, CFOs and Treasurers are stepping up investment in new technologies and hiring talent in new disciplines such as data analytics.
While new technologies and increased data analytics capabilities have the potential to transform the way treasury and finance teams forecast cash, the underlying forecasting methods are often still built on traditional statistical models.
Most cash forecasts are created on a future “Time Series” that bares a close resemblance to a historic time series. Time series forecasts are created by capturing patterns in historic data and extrapolating these patterns into the future. There are a broad range of time series forecasting methods that businesses use today.
Some of the most widely used statistical methods of forecasting are:
Method 1 – Naïve Forecasting
A naïve cash forecast is one that simply uses actual cash flow data for a previous period as the forecast for the upcoming period.
Naïve forecasts, or historical data rollovers, are often used as the starting point for a forecast which is then adjusted for changes in underlying business fundamentals, such as growth or seasonality.
Unadjusted Naïve forecasts are often used for comparison to business cash forecasts which have been created using different techniques, such as direct or indirect forecasting.
Method 2 – Simple Moving Average Forecasting
A simple moving average cash forecast adds cash movements or cash positions, such as net cash inflows of closing cash balances, at points in time for a set period and divides the sum of all numbers by the number of points in time.
The simple moving average method can be useful for forecasting trends. The duration and granularity of the forecast will determine the how many points in time should be used for the forecast. For example, a 30-day simple moving average would be effective for forecasting a trend 30 to 90 days into the future.
Method 3 – Exponential Smoothing
Exponential smoothing can be used to create a cash forecast when the near past is more indicative of the future than the distant past. This method applies decreasing weights to data points over time.
This method of forecasting is particularly useful for creating short-term cash forecasts due the extra weight it applies to the recent past. It is particularly useful and powerful when actual cash flow data is updated on a regular basis.
Method 4 – Box Jenkins
The Box Jenkins method of cash forecasting applies autoregressive moving average (ARMA) and autoregressive integrated moving averages models (ARIMA) to find the best fit for a historic cash flow data set.
These models identify patterns in time (autocorrelations) and are therefore more suitable for longer term forecasting using stable historic data sets.
How to select the appropriate statistical method for your cash forecasts
While deciding which method to use for cash forecasting is, to a certain extent, subject to trial and error, it is always best to consider the business objectives at the outset.
Clarifying the following will provide a valuable baseline for selecting and relying upon a new forecasting method:
- What the forecast will be used for – the business use
- The required duration of the forecast
- The level of detail and granularity required
- How frequently is will be refreshed
- How the output will be summarised and communicated
Then, when a clean historic cash flow or balance data set is in place, running experiments to understand which method works best is reasonably straightforward.
Using software to get started
As mentioned at the start of this article, technological software solutions have the capacity to transform the way treasury and finance teams forecast cash.
While the underlying mathematics of the forecasting models remain the same statistical techniques familiar today, it is the surrounding activity and processes that are revolutionised.
By using software to automate cash forecasting processes, much of the administrative burden can be removed. This therefore dramatically decreases the time required to produce cash forecasts while reducing risk and improving accuracy through the decreased potential for human error.
In addition, sophisticated software tools offer advanced data visualisation techniques so that trends in the data can be identified, providing the level of insight that adds real value back to the core business.
About CashAnalytics
CashAnalytics has helped many companies across a broad range of industries to build and maintain best-in-class cash forecasting processes that leverage software to produce the highest quality reporting and analytics outputs.
If you would like to see a demonstration of how software and automation can improve your cash forecasting processes, or would like to see the business case for introducing CashAnalytics to your company, please contact us directly.